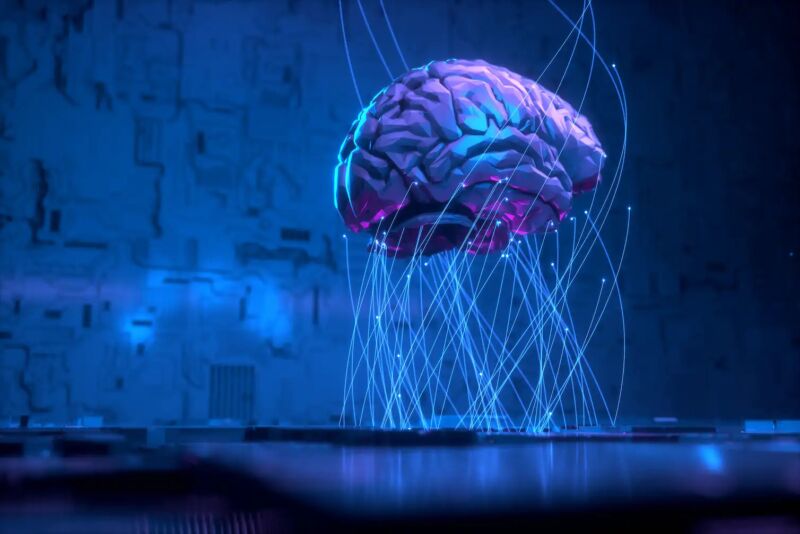
Just_Super/E+ via Getty
Last month, we witnessed the viral sensation of several egregiously bad AI-generated figures published in a peer-reviewed article in Frontiers, a reputable scientific journal. Scientists on social media expressed equal parts shock and ridicule at the images, one of which featured a rat with grotesquely large and bizarre genitals.
As Ars Senior Health Reporter Beth Mole reported, looking closer only revealed more flaws, including the labels “dissilced,” “Stemm cells,” “iollotte sserotgomar,” and “dck.” Figure 2 was less graphic but equally mangled, rife with nonsense text and baffling images. Ditto for Figure 3, a collage of small circular images densely annotated with gibberish.
The paper has since been retracted, but that eye-popping rat penis image will remain indelibly imprinted on our collective consciousness. The incident reinforces a growing concern that the increasing use of AI will make published scientific research less trustworthy, even as it increases productivity. While the proliferation of errors is a valid concern, especially in the early days of AI tools like ChatGPT, two researchers argue in a new perspective published in the journal Nature that AI also poses potential long-term epistemic risks to the practice of science.
Molly Crockett is a psychologist at Princeton University who routinely collaborates with researchers from other disciplines in her research into how people learn and make decisions in social situations. Her co-author, Lisa Messeri, is an anthropologist at Yale University whose research focuses on science and technology studies (STS), analyzing the norms and consequences of scientific and technological communities as they forge new fields of knowledge and invention—like AI.
The original impetus for their new paper was a 2019 study published in the Proceedings of the National Academy of Sciences claiming that researchers could use machine learning to predict the replicability of studies based only on an analysis of their texts. Crockett and Messeri co-wrote a letter to the editor disputing that claim, but shortly thereafter, several more studies appeared, claiming that large language models could replace humans in psychological research. The pair realized this was a much bigger issue and decided to work together on an in-depth analysis of how scientists propose to use AI tools throughout the academic pipeline.
They came up with four categories of visions for AI in science. The first is AI as Oracle, in which such tools can help researchers search, evaluate, and summarize the vast scientific literature, as well as generate novel hypotheses. The second is AI as Surrogate, in which AI tools generate surrogate data points, perhaps even replacing human subjects. The third is AI as Quant. In the age of big data, AI tools can overcome the limits of human intellect by analyzing vast and complex datasets. Finally, there is AI as Arbiter, relying on such tools to more efficiently evaluate the scientific merit and replicability of submitted papers, as well as assess funding proposals.
Each category brings undeniable benefits in the form of increased productivity—but also certain risks. Crockett and Messeri particularly caution against three distinct “illusions of understanding” that may arise from over-reliance on AI tools, which can exploit our cognitive limitations. For instance, a scientist may use an AI tool to model a given phenomenon and believe they, therefore, understand that phenomenon more than they actually do (an illusion of explanatory depth). Or a team might think they are exploring all testable hypotheses when they are only really exploring those hypotheses that are testable using AI (an illusion of exploratory breadth). Finally, there is the illusion of objectivity: the belief that AI tools are truly objective and do not have biases or a point of view, unlike humans.
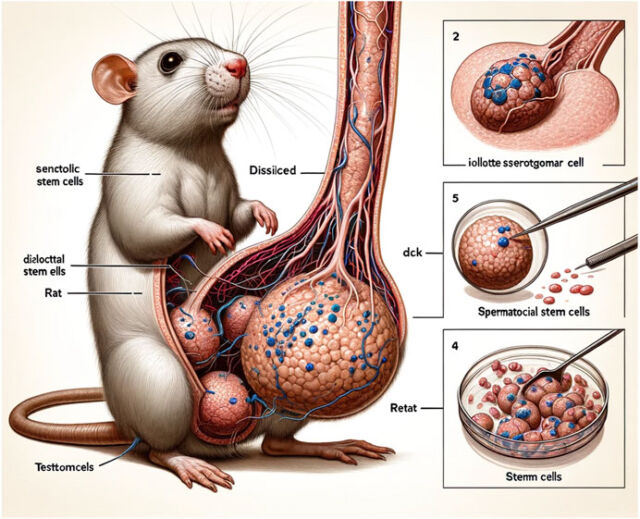
The paper’s tagline is “producing more while understanding less,” and that is the central message the pair hopes to convey. “The goal of scientific knowledge is to understand the world and all of its complexity, diversity, and expansiveness,” Messeri told Ars. “Our concern is that even though we might be writing more and more papers, because they are constrained by what AI can and can’t do, in the end, we’re really only asking questions and producing a lot of papers that are within AI’s capabilities.”
Neither Crockett nor Messeri are opposed to any use of AI tools by scientists. “It’s genuinely useful in my research, and I expect to continue using it in my research,” Crockett told Ars. Rather, they take a more agnostic approach. “It’s not for me and Molly to say, ‘This is what AI ought or ought not to be,'” Messeri said. “Instead, we’re making observations of how AI is currently being positioned and then considering the realm of conversation we ought to have about the associated risks.”
Ars spoke at length with Crockett and Messeri to learn more.
+ There are no comments
Add yours